Choice is a cornerstone of the human experience. Whether it be a life altering decision or as banal as what to have for lunch that day, our lives can be mapped out into a series of choices, twists and turns of this or that. And who has ever had more choices than those of us existing today? With the internet at our fingertips, our options have grown exponentially. One area where the amount of choice has exploded to an almost overwhelming degree, is streaming services.
Challenges
The amount of entertainment available with the click of a button is truly a marvel when one stops to think about it, but if you think about it for too long, that marvel can become quite overwhelming. On Netflix alone, users have access to over 4000 movies and more than 1500 shows. One would think this means a lifetime supply of entertainment and that no user should ever be at a loss for something to watch. But as any Netflix user knows, this is very much not the case. The inability to find something to watch in the vast sea of streaming content seems to often stem from two main issues; there is nothing of interest or there are too many things of interest, and it is impossible to choose. On its face, the idea of a streaming service might seem like a very simple idea; put all the content in one place and let people go find what they want. But as most users know, it doesn’t matter how many shows a platform has if none of it is relevant or interesting to you and if it is difficult to find the shows that are.
Painpoints
Bombarding users with 4000 movies is unlikely to bring anyone back to the platform, but the question then becomes, how to make sure the correct people get pitched the correct show options? This is where AI plays a pivotal role in the success of streaming platforms. Platforms such as Netflix, Hulu, and Peacock make use of AI, specifically machine learning, to create a more enjoyable and less overwhelming experience for their users. Every time a user watches a show, makes a selection, searches for a new movie; the platforms are gathering the data and using it to teach their AI what each user wants to see. One of the most notable examples of this is Netflix’s hyper-specific categories that get pitched to users based on their show selections. Going past the basics of general genre categorization, Netflix users often find themselves logging in to categories such as “female directors behind the camera,” and “TV shows with witty banter.” Many Netflix users are brought to the platform by shows they already know and love. These categories are trying to take those subscribers and keep them on the platform by offering them more shows that are new enough to pique interest but similar enough to shows they love to keep them hooked.
Solutions
Getting a user to take a chance on a new show can sometimes be quite difficult, especially when there are so many choices for them to choose from. AI plays a role in this as well, and is part of the process of creating the trailers that play for users on their home page and on the pages of each show and movie. Many of our choices and decisions in the virtual landscape are made almost instantaneously. Choosing which way to swipe on a dating app or when to stop scrolling through Netflix movies usually comes down to a judgment based on a quick overview of a profile or movie. Along with categorizing content, the AI used by streaming platforms is constantly learning what viewers are seeking in order to create trailers and teasers that will pull them in and get them to stop scrolling through the options.
A lot of focus gets put on the algorithm that makes these choices and categorizations, but the core of the AI framework is the data used to teach these algorithms. Each choice a user makes provides pieces of data that help make their experience on the streaming platform more specific to them.
The importance of quality data in machine learning and training AI cannot be overstated. As streaming platforms grow, the demand for services that create individualized experiences for each user increase as well. The problem that AI developers can often run into is a lack of quality data available to be used in the machine learning process. This is a problem that MagicHub seeks to solve.
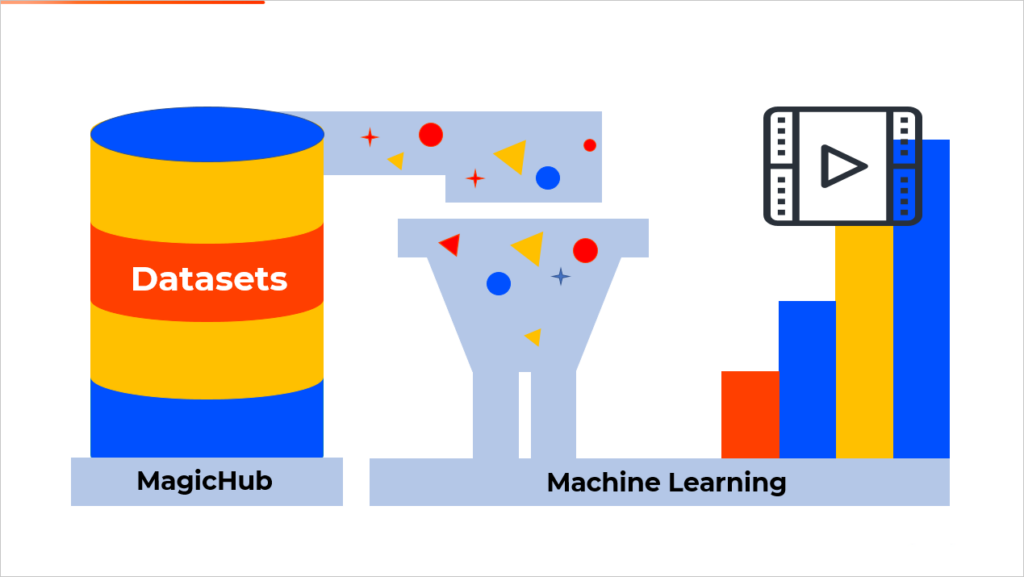
About Magichub
MagicHub is an open-source community that provides access to high quality data sets and an environment where machine learning engineers can experiment, and problem solve. Progress in AI development does not exist without progress in data processing and annotation. A data-centric approach with a focus on finding high quality data to train an AI sets AI developers up with a strong foundation to build their algorithms on. High quality data may not guarantee a successful algorithm, but low-quality data can all but guarantee an unsuccessful AI. When it comes to streaming platforms, data plays a role in every facet of the user experience. From helping to decide what shows are recommended, making sure the subtitles are accurate, and allowing users to voice search for their favorite shows, data is the foundational piece that makes each of these runs smoothly. MagicHub provides users with quality data that can be used to further the development of AI used within these streaming platforms. With data available in many different languages and for a variety of situations, MagicHub seeks to provide machine learning engineers with the resources they need to develop successful algorithms. One of the major strengths of the platform is the amount of conversational data sets available. For more information about the MagicHub community and the data sets available for more specific needs, find us at https://www.magichub.com.