As of 2021, the adoption rate of intelligent voice interaction functions in Chinese passenger cars has reached 86%. At present, the car cockpit is trending toward intelligent development, and the in-vehicle voice assistant is the core function of the intelligent cockpit. Since the driver's hands and eyes are occupied by tasks during the driving process, the in-vehicle scene has higher requirements for the hands-free interaction voice function.
Recently, major companies have been rushing to invest in the field of intelligent vehicle voice interaction. Xiaodu in cooperation with NIO will optimize and upgrade the experience of using NIO's in-vehicle voice operating system to further enhance the human-vehicle interaction experience. Not long ago, Microsoft officially announced that with the support of the deep neural network TTS (Text-to-Speech) based on Microsoft's intelligent cloud Azure, China's leading intelligent electric vehicle company Xiaopeng Motors has successfully completed the upgrade of its vehicle-grade voice assistant. Huawei has even begun to independently develop smart cockpits. For most companies, the smart car voice assistant is the biggest selling point of the current smart cockpit development. Today, let's talk about the challenges and solutions faced by the development of intelligent in-vehicle voice assistants.
CHALLENGE
Intelligent voice assistants are widely used. Among them, automobiles, as the most special application scenario, are naturally different from other scenarios, so they are also more challenging.
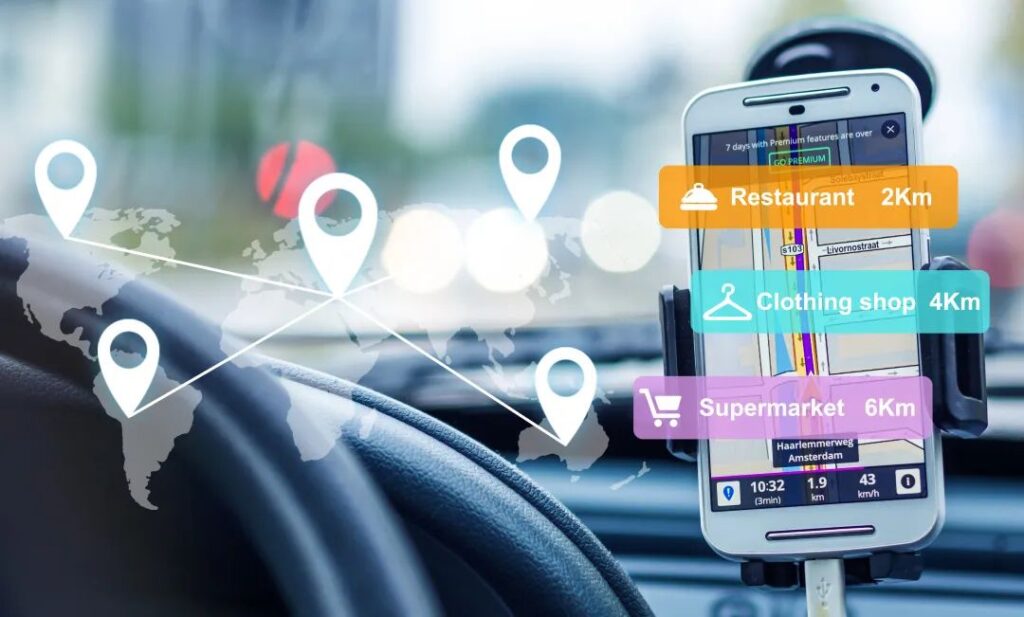
Challenge #1: the particularity of the driving scenario, brings a series of difficulties to the vehicle voice interaction.
Specifically, in a very complex acoustic environment such as a car, the car voice assistant faces the challenges of noise interference, severe reverberation, multi-person voice aliasing, various wind, rain, and other vehicle noise interference outside the car. The accurate recognition of speech, the enhancement of speech quality, and the high-quality speech interaction are all relatively difficult issues. At the same time, in-vehicle voice assistants may involve privacy issues, which are also challenges that need to be faced to consumers.
Challenge #2: There are many restrictions on in-vehicle hardware devices and higher requirements for model and interaction accuracy
In order to cope with the strict standards of the vehicle level, the model size and real-time rate of the in-vehicle voice system need to be controlled within a low range, the CPU usage is low, and the overall response time is fast. Compared with ordinary recognition models, the indicators have higher requirements.
Challenge #3: The lack of data in the vehicle voice scene is the bottleneck of current research and implementation
At present, the data accumulation for in-vehicle AI voice training is still insufficient, and semantic understanding and verification in driving scenarios still need to be improved. Although the interaction data of devices such as smart speakers and robots can provide certain data support for the in-vehicle scene, they cannot completely replace the data of the in-vehicle scene interaction.
SOLUTION
Option 1: Make the in-vehicle voice assistant smarter with in-vehicle voice data that matches the actual scene
With more mass production of voice assistants, the corresponding technical research can change from a priori to a data-driven one. Using the actual scene recording data to train the intelligent voice assistant model can make it smarter and smarter, and reduce the recognition rate drop caused by data mismatch. At the same time, in the process of using the intelligent in-vehicle voice assistant, the user can also fine-tune the voice assistant model through the application of local data, so that the model becomes more and more intelligent, and the recommended services become more and more in line with the user's needs.
Option 2: Algorithm research reduces noise, reduces model size, and improves recognition accuracy
The process involved in in-vehicle voice interaction is cumbersome, involving everything from linguistics to acoustic theory, and at the same time, it needs to be adapted to special driving scenarios. In the application process of voice interaction in the car, ASR (including signal input, noise reduction and phoneme selection and other processes), NLP (including NLU and NLG, involving part-of-speech tagging and text information processing), TTS (including back-end splicing and synthesis of speech), which is also the core link of voice personification) has become three key links. How to link each link ingeniously, assist each other, and build a complete and smooth overall algorithm framework is the current focus of academia and industry. In fact, the algorithm driving is also inseparable from the assistance of the actual vehicle voice data, because the key to solving the difficulty of the vehicle voice assistant is the recording of the vehicle voice data in the actual scene.
No matter how excellent the in-vehicle voice assistant technology route is, it is inseparable from the upstream in-vehicle data. As the world's leading provider of AI data solutions, Magic Data empowers enterprises with high-quality datasets and solutions. At present, Magic Data has provided in-vehicle voice data in multiple languages and mixed languages for many automobile industry enterprises and voice interaction system R&D enterprises. The in-vehicle scene data includes multiple languages, multiple noise environments, and multi-device recordings, etc.
Recommendations
Guangzhou Cantonese In-Vehicle Speech Corpus—Smart Mobility
Thai In-Vehicle Scripted Speech Corpus—Smart Mobility
Visit www.magicdatatech.com for more information.